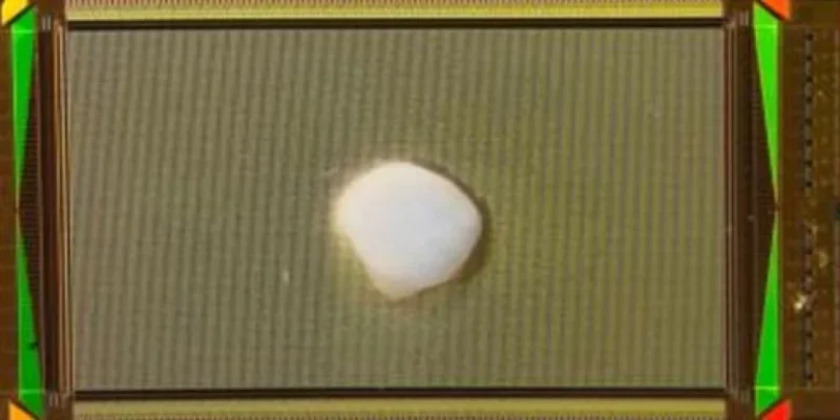
Scientists have managed to create a biocomputer by integrating the human brain with electronics.
There is no computer even remotely as powerful and complex as the human brain. The lumps of tissue in our skulls can process information at a rate and speed that computers can barely match. The key to the brain’s success is the efficiency of the neuron as both a processor and a memory device, as opposed to physically separated units in most modern computer devices. There have been many attempts to make computing more brain-like, but a new attempt goes one step further by integrating real, actual human brain tissue with electronics.
It’s called Brainoware, and it works. The team, led by engineer Feng Guo from Indiana University in Bloomington, set him tasks such as speech recognition and prediction of nonlinear equations. It was slightly less accurate than a hardware computer powered by artificial intelligence, but the research demonstrates an important first step in a new type of computer architecture. However, while Guo and his colleagues followed ethical principles in developing Brainoware, several researchers from Johns Hopkins University note in a commentary in Nature Electronics the importance of keeping ethical considerations in mind as the technology is further expanded.
Lena Smirnova, Brian Caffo and Eric K. Johnson, who were not involved in the study, caution: “As the complexity of these organoid systems grows, it is imperative that the community examine the many neuroethical issues that surround biocomputer systems that include human nervous tissue.”
The human brain is incredibly amazing. It contains approximately 86 billion neurons on average and up to a quadrillion synapses. Each neuron is connected to 10,000 other neurons that are constantly working and communicating with each other.
To date, our best attempts to model brain activity in an artificial system have barely scratched the surface. In 2013, Riken’s K Computer, then one of the world’s most powerful supercomputers, attempted to simulate the brain. With 82,944 processors and a petabyte of main memory, it took 40 minutes to simulate one second of activity of 1.73 billion neurons connected by 10.4 trillion synapses – roughly only one to two percent of the brain.
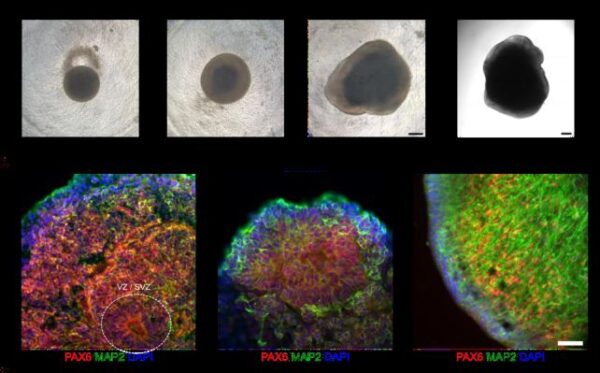
In recent years, scientists and engineers have been trying to get closer to the brain’s capabilities by developing hardware and algorithms that mimic its structure and how it works. Known as neuromorphic computing, it is improving, but it is energy-intensive, and training artificial neural networks takes a long time. Guo and his colleagues sought a different approach, using real human brain tissue grown in the laboratory. Human pluripotent stem cells were forced to develop into different types of brain cells, which organized into three-dimensional mini-brains called organoids, complete with connections and structures.
These are not real brains, but simply tissue formations without any resemblance to thought, emotion, or consciousness. They are useful for studying how the brain develops and works without digging into a real person.
Brainoware consists of brain organoids connected to an array of high-density microelectrodes using a type of artificial neural network known as reservoir computing. The electrical stimulation transfers information to the organoid, the reservoir in which this information is processed before Brainoware releases its calculations in the form of neural activity. For the input and output levels, ordinary computer hardware is used. These layers had to be trained to work with the organoid, and the output layer read the neural data and made classifications or predictions based on the input data.
To demonstrate the system, the researchers provided Brainoware with 240 audio recordings of eight male speakers making Japanese vowel sounds and asked it to identify the voice of one particular person. They started with a naive organoid; after only two days of training, Brainoware was able to identify the speaker with 78 percent accuracy.
They also asked Brainoware to predict the Henon map, a dynamic system that exhibits chaotic behavior. They left it unattended to learn for four days – each day representing an epoch of training – and found that it was able to predict the map with better accuracy than an artificial neural network without a long block of short-term memory.
Brainoware was somewhat less accurate than artificial neural networks with long short-term memory, but each of these networks had gone through 50 epochs of training. Brainoware achieved similar results in less than 10 percent of the training time.
“Due to the high plasticity and adaptability of organoids, Brainoware has the flexibility to change and reorganize in response to electrical stimulation, which highlights its ability to perform adaptive backup computing,” the researchers write.
There are significant limitations, including the problem of preserving live and healthy organoids, as well as the power consumption levels of peripheral equipment. But ethical considerations aside, Brainoware is not only important for computing, but also for understanding the mysteries of the human brain.
“It may be decades before general bio-computing systems can be created, but this research is likely to provide fundamental insights into the mechanisms of learning, neural development, and cognitive consequences of neurodegenerative diseases,” Smirnova, Caffo, and Johnson write.
“It can also help develop preclinical models of cognitive impairment for testing new therapeutics.”